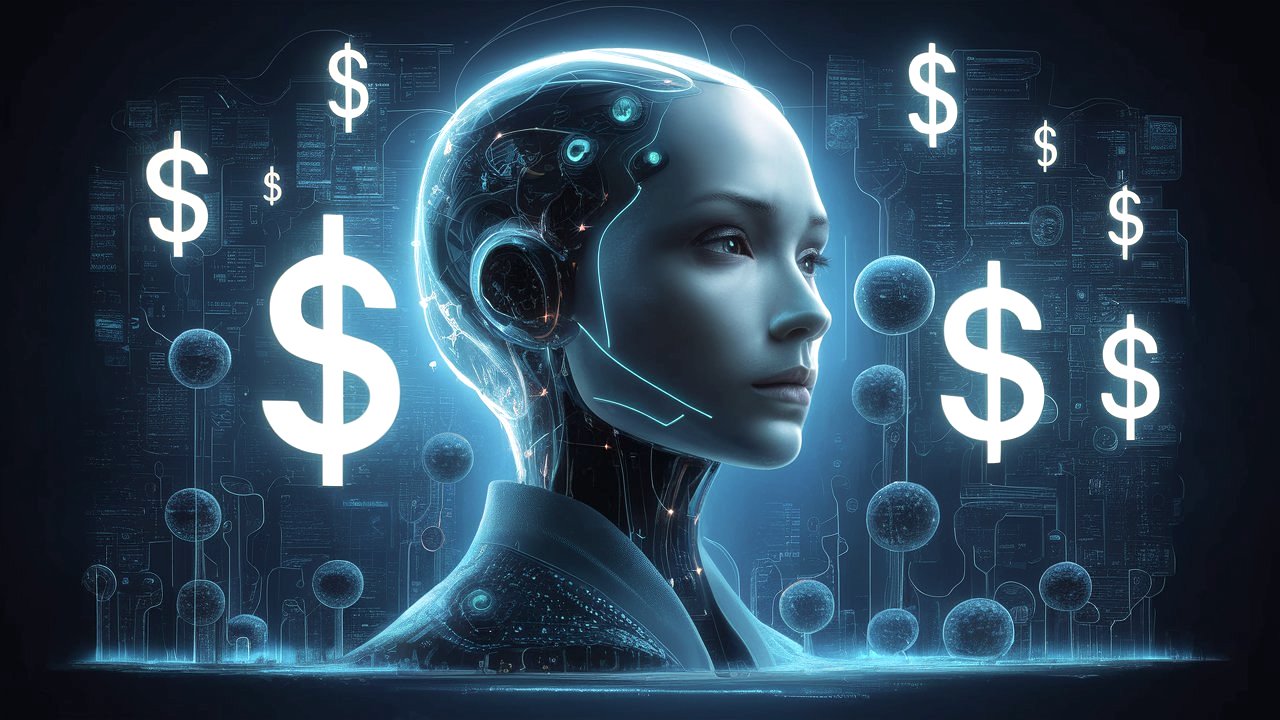
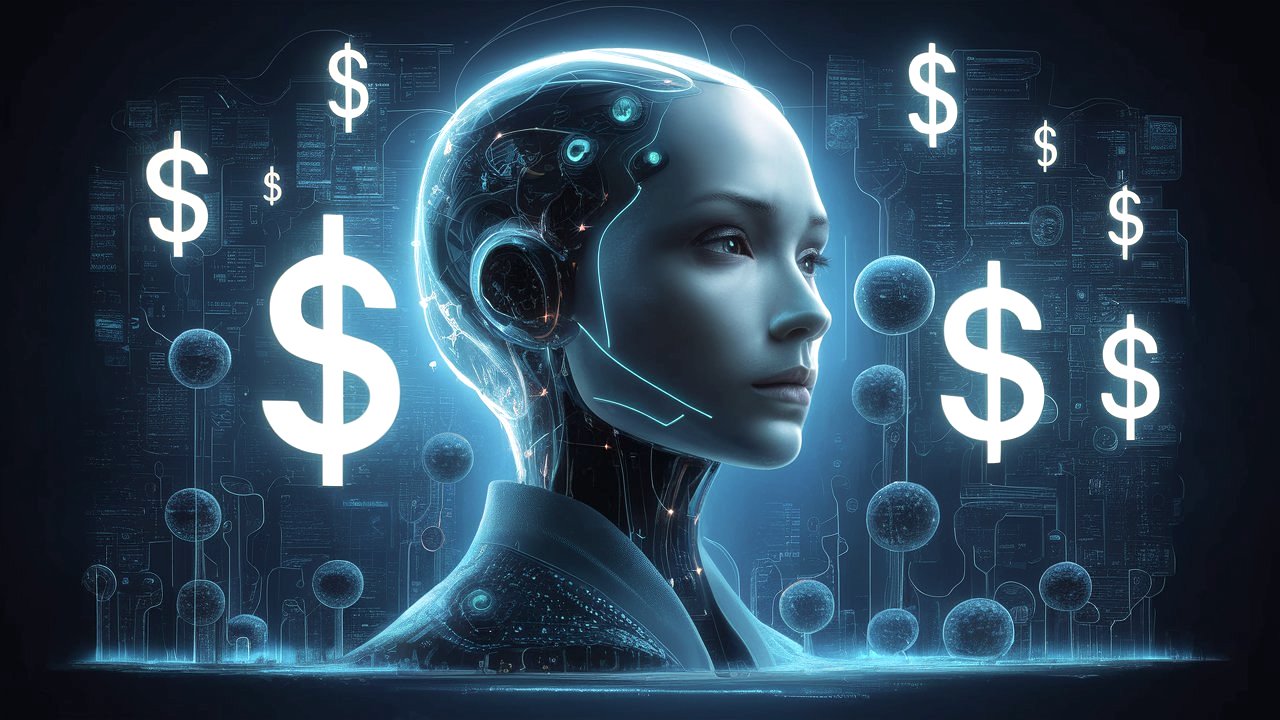
Generative artificial intelligence models are at the heart of the AI revolution today. As enterprises expand their gen AI initiatives, they recognize that the cost of developing, deploying and operating these models can be significant as use cases scale and expand.
Organizations transitioning from gen AI pilots to production experience a rude awakening when it comes to costs. Creating a production-ready gen AI system can be orders of magnitude more expensive than running a pilot.
Organizations can take advantage of the following five best practices to optimize gen AI costs. Implementing these best practices allow organizations to maximize the return on their gen AI investment and unlock its full potential.
Selecting the appropriate model often involves making the right tradeoffs between several factors, with model accuracy, performance and costs being the most significant ones. The size of a gen AI model (measured by the number of its training parameters) has significant bearing on these metrics. Though larger gen AI models deliver higher accuracy, they often come with higher costs and latency in model responses.
Selecting the right model must be a multidimensional evaluation process. Model accuracy needs to be validated through a broad set of accuracy metrics such as fluency, coherence, relevancy and contextual understanding. If choosing a gen AI model delivered as an applied programming interface, remember that the same model may be offered by multiple providers. This enables organizations to choose a provider that delivers superior price and performance, yet meets the security and support needs of the organization.
A great way to enable safe experimentation is to create an AI model garden with multiple models being made available to users and developers. An AI model garden features available models in a self-service manner as part of a model catalog, underpinned by basic security and privacy principles. Early adopters make gen AI models available from more than one provider and often mix and match models in the model catalog. This makes both large and small AI models available, while ensuring the availability of open-source models alongside closed-source ones.
Information technology leaders should create an AI model garden and offer multiple, diverse models for users to safely experiment. Make the model costs transparent to the users via reporting tools, which enables them to make better economical choices without jeopardizing their accuracy, performance and other selection metrics.
When augmenting and customizing gen AI models, businesses need to weigh upfront and running costs. Upfront costs are dedicated to the selection of different approaches, encompassing model augmentation such as prompt engineering and retrieval-augmented generation, or RAG, and model customization such as fine-tuning and training a model from scratch — each increasing in complexity and cost.
Running costs can be mitigated by careful choice of models that balance price/performance, or even by efficiently fine-tuning a model on a specific dataset through instruction tuning or continuing pretraining. This is notable, as it could reduce the need for additional text via prompt engineering or RAG.
IT leaders should consider augmentation and customizations sequentially, only moving to a more advanced approach if a simpler one doesn’t meet the required output quality. They can evaluate the different approaches not only to achieve better output quality, but also to reduce running costs — especially if the model usage will be high volume and predictable.
Self-hosting gen AI models (often on-premises) can seem attractive for businesses seeking increased control and data privacy. It is also true that model inference will be more hybrid in the future, driven by costs, performance and privacy needs. However, it’s crucial to be aware of the potential tradeoffs, as the list of cost drivers for self-hosting is extensive.
Consider the complexity and cost implications before opting to self-host gen AI models. If an organization decides to self-host, it must ensure it can deliver opex-based pricing models or managed services for it. IT leaders should evaluate their organization’s capacity for upfront investment, ongoing maintenance and expertise before opting for self-hosting, considering that the costs and complexities can escalate — especially with larger models and high usage volumes.
Prompt design involves crafting prompts that effectively evoke the desired responses from gen AI models. Crafting well-structured prompts is crucial for guaranteeing precise, top-notch outputs. Prompt design is important since it has a significant implication on the accuracy and relevancy of model responses, adaptability of the models to specific tasks and, most importantly, on model inference costs. Well-crafted prompts can ensure model responses are concise, relevant and accurate, and are generally a cheaper way to steer AI models.
Explore prompt design tools that can boost the quality of prompting and save money in the long run. Document these best practices and encourage wider dissemination through knowledge sharing sessions.
Organizations should analyze and uncover hidden gen AI costs across various approaches. Avoid expensive model customizations and understand the tradeoffs of self-hosting AI models. Lower model inference costs though techniques such as prompt design. Conduct monthly or quarterly reviews of gen AI costs to ensure ongoing optimization and instill a culture of accountability.
Arun Chandrasekaran is a distinguished VP analyst at Gartner Inc., where he researches emerging technologies and trends, with an emphasis on artificial intelligence and cloud computing. He wrote this article for SiliconANGLE.
THANK YOU